Predictive Lead Scoring: Your Smartest Sales Move Yet
Updated on
By Bradley Kovacs
Bradley Kovacs
Bradley has been passionate about technology since childhood, starting with Microsoft Flight Simulator at age six. In college, he automated his data e...
learn more
Bradley Kovacs
Bradley has been passionate about technology since childhood, starting with Microsoft Flight Simulator at age six. In college, he automated his data e...
Table of Contents
Table of Contents
Sales teams love a good lead, like, really love them. But chasing the wrong ones? That's a heartbreak your pipeline can't afford.
Fortunately, it doesn't have to be hard anymore because of the onset of predictive lead scoring, the smarter, effective cousin of the old-school gut-feel approach. It's not magic, but it does feel pretty close when algorithms, machine learning, and data-driven insights start serving up leads that actually convert.
We're talking about going beyond basic demographics and behavior tracking. This is where things get advanced, where your CRM starts whispering, "Hey, this one's a hot prospect."
Whether you're using predictive lead scoring in your marketing automation system, experimenting with machine learning models, or just figuring out what predictive lead scoring even is, you're about to level up your lead game.
This in-depth guide will explain how predictive lead scoring works, why it matters, and how your sales team can stop guessing and start closing.
You're on the right track, so stick around.
How Predictive Lead Scoring Works
So, how exactly does your CRM know who's a lead and who's just lurking?
Here's the deal: predictive lead scoring takes a ton of data, runs it through machine learning algorithms, and pops out a score that tells you how likely someone is to convert.
The Data Inputs
Every intelligent prediction starts with the correct data. Predictive lead scoring pulls from several sources to get the complete picture of who your leads are and how they behave. Here's what goes into the mix:
- Demographics: This includes details such as job title, industry, location, and company size. Someone in a decision-making role at a mid-sized company? Way more promising than a student just browsing. Demographics help the algorithm filter who might be a good fit.
- Behavioral: Clicks, page visits, downloads, email opens, you name it. Behavioral data tells you how engaged a lead is. If someone's visiting your pricing page five times in two days, that's not just a coincidence. Predictive models weigh this heavily.
- Firmographic: Think of this as business demographics. Revenue, number of employees, and tech stack. These factors help determine how qualified a company is. B2B lead scoring leans hard on firmographic data to qualify leads that align with your ideal customer profile.
- CRM Interactions: Every form filled, webinar signup, and support ticket logged in your CRM is a breadcrumb. Predictive lead scoring in HubSpot or any other CRM uses this data to connect the dots between past behavior and future potential.
The Scoring Model: How AI Assigns Probability to Convert
This is where the magic happens (okay, it's machine learning, but it feels like magic). The predictive model examines historical data to determine who became a customer and who didn't, and then uses predictive analytics to identify patterns. From there, it builds a model that ranks new leads based on how closely they match those success patterns.
For example, let's say leads who attend a product demo and are in the Saas industry convert at a 32% higher rate. The algorithm takes note. Then, next time a SaaS lead books a demo? They'll get a higher predictive lead score.
Bonus: The more data you feed it, the smarter it gets. That's where predictive lead scoring machine learning stands out. It keeps learning, adapting, and getting sharper with every interaction.
Differences Between Rules-Based and Predictive Models
Not all lead scoring methods are the same. While rules-based lead scoring is the traditional "if-this-then-that" model, predictive lead scoring is a lot more dynamic and data-driven.
Here's how they stack up:
Feature |
Rules-Based Lead Scoring |
Predictive Lead Scoring |
Setup |
Manual setup of scoring criteria. |
Automated, based on machine learning. |
Data Used |
Limited (basic demographic & behavior). |
Rich data sets (demographic, firmographic, CRM). |
Flexibility |
Rigid, requires constant manual updates. |
Adaptive, learns and updates automatically. |
Accuracy |
Lower, based on assumptions. |
Higher, based on real data patterns. |
Time to Implement |
Faster to set up. |
Takes longer to train, but worth it. |
Scalability |
Not ideal for high lead volume. |
Perfect for scaling sales teams. |
Rules-based models are fine if you're just getting started. But if you're handling a high volume of leads or want to stop manually tweaking scores every week, advanced predictive lead scoring is where you want to be.
Why Sales Teams Need Predictive Lead Scoring Now
Sales reps have one major enemy: wasted time. Between chasing cold leads and manually qualifying every inquiry, there's a ton of energy being poured into people who were never going to convert.
That's why predictive lead scoring isn't just a nice-to-have anymore. It's a must! Allow us to explain why below.
Stats on Lead Conversion Rates and Time Spent on Unqualified Leads
Here's a reality check: According to Gartner, only 23.9% of sales emails are ever opened. And, depending on your industry, the average lead-to-customer conversion rate ranges from 2% to 10%. That's a whole lot of hustle for a pretty modest payoff.
With predictive lead scoring, you can cut through the noise and focus on leads that are worth pursuing. Instead of casting a wide net, your team can laser-focus on leads who are more likely to make a purchase, saving time, energy, and the company's budget.
Shift Toward Automation and Smarter Systems
More modern businesses are embracing automation and intelligent systems to streamline processes and gain a competitive edge. Sales is no exception. Teams are recognizing the limitations of manual processes and the power of data-driven insights.
Predictive lead scoring, often powered by machine learning, perfectly aligns with this shift. It automates the usually tedious and subjective task of lead prioritization, providing a data-backed, objective assessment of each lead's potential.
This move towards smarter systems allows sales teams to operate more strategically, making informed decisions based on probabilities rather than hunches. Integrating tools like a lead scraper to gather potential leads efficiently and then using predictive scoring to prioritize them creates a powerful synergy.
Furthermore, a well-implemented predictive scoring system complements effective lead-nurturing strategies by ensuring that the right leads receive the proper engagement at the right time.
Overview of Industries Benefiting the Most
Some industries are already seeing major wins from predictive models. Here's where predictive lead scoring is turning heads (and boosting conversions):
- Insurance: In the insurance industry, predictive lead scoring helps identify individuals who are most likely to purchase policies based on factors like demographics, life events, and previous interactions. This allows agents to focus on prospects who are actively in the market for insurance products, resulting in higher conversion rates and a more efficient use of their time.
- SaaS: For SaaS companies, predictive lead scoring machine learning algorithms can analyze user behavior during free trials, engagement with marketing materials, and company demographics to pinpoint leads with the highest propensity to subscribe. This enables sales teams to prioritize follow-ups with these high-potential users.
- Real Estate: Predictive lead scoring in real estate can analyze factors like budget, preferred location, property type, and engagement with online listings to identify serious buyers. This allows agents to focus their efforts on clients who are most likely to make a purchase, streamlining the sales process and maximizing their success.
These are just a few examples, and the benefits of predictive lead scoring extend across numerous other industries seeking to optimize their sales processes and drive revenue growth.
The Role of Predictive Analytics in Lead Scoring
At the heart of predictive lead scoring is predictive analytics, the tech that crunches numbers, spots patterns, and tells your CRM who's ready to convert. You don't need to be a data scientist to get how it works, but knowing the basics can help you see why it's such a game-changer for sales.
Overview of Predictive Analytics
Let's keep this simple: predictive analytics looks at what has already happened to make intelligent predictions about what is likely to happen next. Think of it as your GPS for sales. It uses past traffic (aka customer behavior) to predict where the next turn should be.
For example, if a lead signs up for a webinar, downloads an eBook, and clicks through a pricing email, predictive analytics detects these signals and says, "Hey, we've seen this combination before—they usually convert."
Instead of assigning points based on gut instinct, lead scoring and predictive analytics assign probabilities based on patterns they've seen across hundreds (or thousands) of similar leads. This application of AI for leads empowers sales teams with foresight, allowing them to focus on the opportunities with the greatest potential.
Use of Historical Data, Patterns, and Trends
Predictive models analyze historical data, including success stories, near-misses, and total duds. Every closed deal, lost lead, or ghosted email becomes part of the model's training set.
From there, the algorithm looks for patterns. Maybe your best leads always:
- Come from companies with 50–200 employees
- Request a demo within one week of visiting the site
- Read two blog posts before opening a pricing email
Those behaviors, once spotted, get weighted in the algorithm. This is the backbone of predictive lead scoring algorithms. They don't just guess who's interested. They know based on data.
As more leads flow through the funnel, the model gets sharper. And with the rise of tools built specifically for AI sales lead generation, it's easier than ever to collect this data and use it in real-time.
How Predictive Analytics Improves Lead Prioritization
Here's where predictive analytics really shines: prioritization.
Instead of your reps staring at a list of 300 leads, wondering who to call first, predictive lead scoring ranks them based on their likelihood to convert. High-score leads go to the top of the call list. Lower-score leads get nurtured until they're ready.
That kind of efficiency pays off. According to research, businesses that use predictive analytics are 2.9 times more likely to report revenue growth above the industry average. Why? Because their teams spend less time guessing and more time selling.
Whether you're dealing with inbound leads or running outbound campaigns, predictive analytics helps ensure you're putting your best effort where it counts most.
Predictive Lead Scoring vs. Traditional Lead Scoring
Predictive lead scoring and traditional methods differ significantly in their approach, sophistication, and effectiveness. Here's how:
Feature |
Traditional Lead Scoring |
Predictive Lead Scoring |
Scoring Mechanism |
Manual assignment of points based on predefined rules. |
Automated scoring based on statistical analysis of data. |
Data Analysis |
Limited to explicitly defined criteria. |
Analyzes a wide range of data points and identifies patterns. |
Automation Level |
Low; requires manual updates and adjustments. |
High; continuously learns and adapts automatically. |
Scalability |
Can become cumbersome with increasing data and rules. |
Easily scales to handle growing data and lead volumes. |
Accuracy |
Potentially lower; prone to subjectivity and bias. |
Generally higher, data-driven and objective. |
Manual Point-Based Systems vs Automated Scoring
Manual lead scoring systems assign values to actions, such as +10 points for a demo request or +5 points for email opens. While this works for basic lead tracking, it's time-consuming and easy to mess up. It relies on your team making the proper judgment calls every time.
Predictive lead scoring, on the other hand, automates this process using historical data and machine learning. It identifies what behaviors lead to conversions, not just what seems essential. The result? Smarter scores that update in real time, not just when someone edits a spreadsheet.
But if you need help building your lead pipeline before you start scoring, here's a guide on how to use AI to generate leads that pairs perfectly with predictive scoring.
Speed, Scalability, and Accuracy Comparison
Manual systems slow down as lead volume grows. Every new action or behavior has to be evaluated and scored. That might be manageable with 100 leads, but with 10,000? Not so much.
Predictive lead scoring thrives at scale. It processes thousands of data points in seconds, delivering real-time prioritization with far better accuracy.
Which One Is Right Depending on Business Stage
The optimal choice between traditional and predictive lead scoring often depends on a business's stage of development and resources:
- Startups or small businesses with limited data can get by with traditional scoring. It's faster to set up and doesn't need a mountain of CRM activity to work.
- Scaling businesses that have a sufficient lead volume and historical data should definitely invest in predictive lead scoring. The ROI grows as your pipeline does.
Implementing a Predictive Lead Scoring System
Transitioning to a predictive lead scoring system requires careful planning and execution. However, the long-term benefits in terms of sales efficiency and revenue generation make the effort worthwhile.
Step-by-Step: From Data Collection to Score Deployment
Implementing predictive lead scoring involves a systematic process:
- Collect and Centralize Your Data: Pull in as much historical lead and customer data as you can. This includes CRM activity, website behavior, email engagement, firmographics, and more.
- Choose Your Predictive Model: Many modern CRMs have built-in predictive lead-scoring algorithms or integrations that support machine learning. Select a platform that fits your business size and complexity.
- Train the Model: The system will analyze your past lead behavior, who converted, who didn't, and find patterns that predict future success. The more quality data you have, the smarter the model becomes.
- Deploy Scoring to Your Team: Once the model is trained, scores are automatically assigned to leads in your CRM system. Reps can instantly prioritize based on conversion likelihood.
- Monitor and Refine: Predictive models improve over time, but you still need to track accuracy, tweak inputs, and stay aligned with evolving sales goals.
Checklist
Here's your quick implementation checklist to make sure nothing slips through the cracks:
- Audit Your Current Data: Remove duplicates, fix errors, and ensure consistency across fields before training your model.
- Choose a CRM or Tool With ML Capabilities: Opt for a platform that supports predictive lead scoring and machine learning.
- Set Up Goal-Tracking and Feedback Loops: Establish what success looks like, conversions, deal size, time to close, and build feedback loops to improve the model's output over time.
- Train Your Team: Ensure your sales reps understand what the scores mean, how to use them, and when to override them with their intuition. The system is smart, but people still close the deals.
Final Thoughts
From saving time to closing more deals, predictive lead scoring empowers sales teams to focus on the right prospects at the right moment. By combining historical data, machine learning, and automation, businesses across industries are turning lead chaos into revenue clarity.
Whether you're running a B2B SaaS company, managing real estate leads, or working in insurance, the benefits of advanced predictive lead scoring are clear: more accuracy, less guesswork, and better sales outcomes.
Want to see it in action? Take the next step and see how Ringy can help you build a smarter, faster, and more effective lead pipeline. Request a demo today and put predictive lead scoring to work for your team.
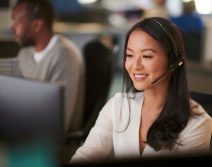
Skyrocket your sales with the CRM that does it all.
Calling? Check. SMS? Check. Automation and AI? Check. Effortlessly keep in touch with your customers and boost your revenue without limits.
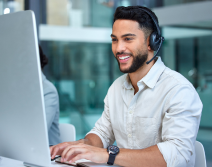
Take your sales to new heights with Ringy.
Sales in a slump? Ringy gives you the tools and flexibility you need to capture leads, engage with them, and turn them into customers.
Subscribe to Our Blog
Enter your email to get the latest updates sent straight to your inbox!